Cybersecurity and AI: Understanding and Mitigating Common Cybersecurity Threats to AI Models
V&E Cybersecurity Update
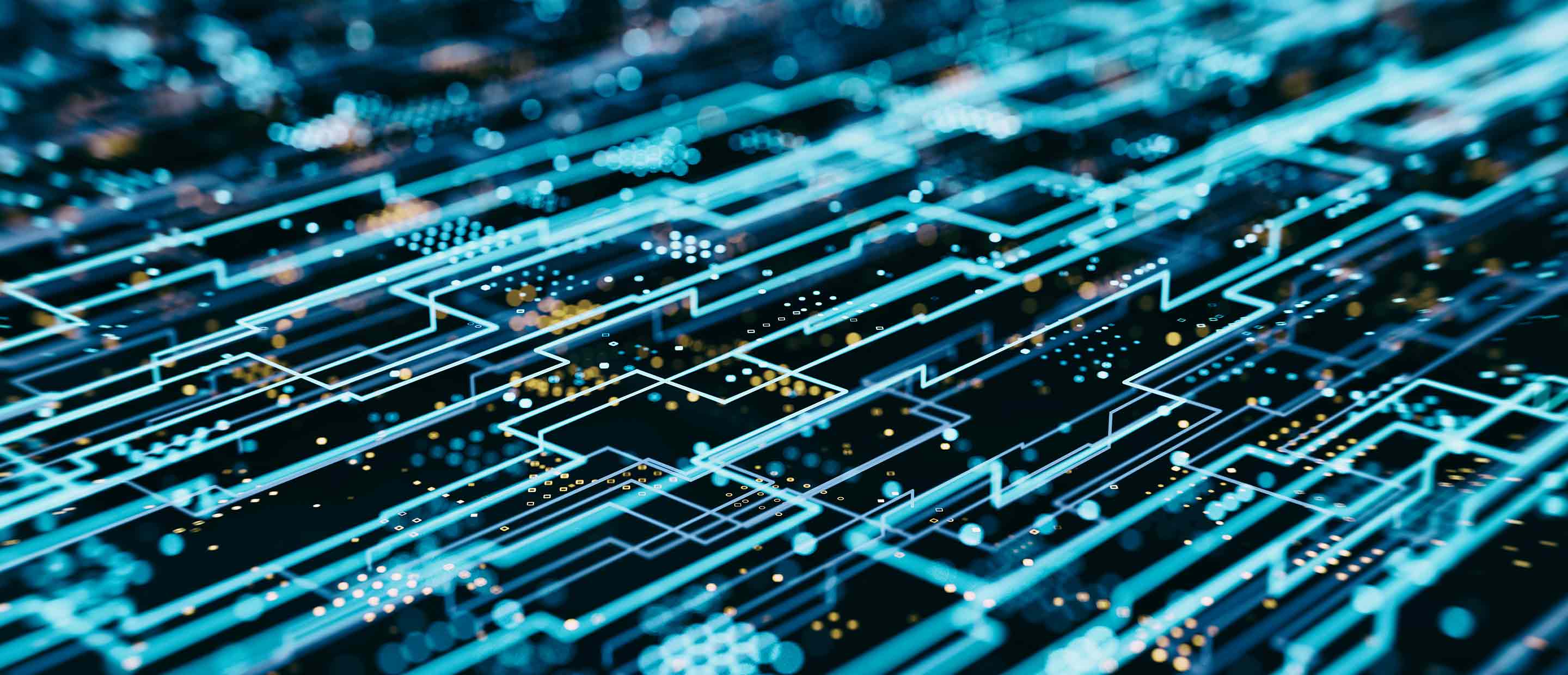
V&E Cybersecurity Update
By Palmina Fava, Parker Hancock, and Dean Dixon
Artificial intelligence (“AI”) is revolutionizing the workplace, and bringing with it unique cybersecurity challenges. With the increased prominence of AI also comes the need for new cybersecurity approaches.
As AI models are increasingly used in critical applications such as finance, health care, and national security, the potential for cyber-attacks targeting these systems is growing. The cybersecurity threats to AI models are new and distinct from more common and understood software exploits. Three of the most prevalent AI cybersecurity threats are data poisoning, evasion attacks, and confidentiality attacks. By understanding the unique vulnerabilities of various AI models, organizations can take steps to ensure the effective implementation of AI systems into their workplace while minimizing business risks.
Data Poisoning
When ChatGPT first launched at the end of November 2022, many users immediately started using it to help solve coding problems. But ChatGPT generates responses based on word association, with no independent fact-checking ability. As programming message boards became inundated with ChatGPT-based answers, users quickly realized the answers were not reliable, and sometimes were shockingly wrong. As a result, ChatGPT was banned from Stack Overflow, one of the most popular programming fora, within one week of ChatGPT’s launch. While ChatGPT’s factual errors were accidental, malicious actors could use similar techniques as part of intentional efforts to spread misinformation. This is what is known as a data poisoning attack.
A data poisoning attack involves the deliberate manipulation of an AI model’s training data to cause the model to make incorrect decisions or to behave in unexpected ways. By injecting malicious or misleading information into the training data, the AI system can learn incorrect patterns or relationships between input data and the target outputs. As many AI models are trained on data gleaned from the internet, planting this malicious data can be as easy as editing a Wikipedia page or planting false information on Reddit. When used in production environments, this poisoned model can produce bad decisions with serious consequences. The potential for harmful consequences only expands as AI systems are entrusted with more critical business applications. These types of attacks can be mitigated by carefully vetting and validating the training data and by monitoring the AI system’s performance over time to detect any potential anomalies or signs of attack.
Evasion
The Defense Advanced Research Projects Agency (“DARPA”) developed an advanced computer-vision AI system to warn soldiers of approaching threats. A group of Marines was challenged to sneak past a security camera equipped with the system. Two Marines avoided detection by hiding under a cardboard box. Another avoided detection by holding a fake tree in front of him. Yes, really. This is an example of an evasion attack.
Evasion is a type of attack in which an attacker manipulates the input data to cause the AI system to make incorrect predictions or decisions. The goal of an evasion attack is to bypass the system’s defenses by crafting input data specifically designed to mislead or to deceive the AI model. For example, an attacker may alter images or manipulate audio data in a way that makes it appear benign to the AI system, when in reality it contains malicious content. This can lead to security breaches or misclassification of data and can undermine the overall effectiveness and reliability of the AI system. In the DARPA example, the model had been trained to recognize walking soldiers, not moving boxes and trees, and thus completely missed the hiding Marines.
To prevent evasion attacks, it is important to design AI systems that are robust and resilient against such manipulation, and to develop testing methods that can identify and address potential weaknesses in the AI model’s ability to accurately classify and process input data.
Confidentiality
You probably don’t know Ann Graham Lotz, but Stable Diffusion does. Stable Diffusion is a now-famous generative AI model for creating images based on text prompts. Researchers realized that Stable Diffusion could recreate images from its training set when appropriately prompted — even when theoretically the model shouldn’t have a copy of that image. This is an example of a confidentiality attack — an attempt to steal, modify, or expose sensitive information processed or stored by the AI system. This type of attack can be initiated by malicious actors who gain unauthorized access to the AI system, by insiders who abuse their privileges to access sensitive information, or even by crafty users who manipulate a system’s inputs to better understand how its outputs are categorized.
Confidentiality attacks can have serious consequences, especially in cases where the AI system handles sensitive information, such as personal identification data, financial records, trade secrets, or intellectual property. If sensitive information is used in model training, it may be possible to recover that information from the trained model.
Mitigating this risk includes carefully considering whether sensitive information is necessary for model training (as opposed to de-identified information), and placing appropriate safeguards on the maintenance and use of the model to prevent exposure of confidential or sensitive information.
What This Means for You
The proliferation of AI technologies brings new cybersecurity threats that should be considered by developers and users of AI models. As AI becomes more widely used in the workplace and as a critical component of applications and workstreams, it is important for organizations to take these threats seriously and to implement the necessary security measures to protect themselves and their new AI systems. Experienced attorneys can play a crucial role in protecting the organization by advising on legal and ethical considerations related to AI security, as well as working with other stakeholders to ensure that the AI systems are designed and deployed in a secure manner.
Vinson & Elkins’s Cybersecurity, Data Privacy, and Technology teams have deep experience on AI, cybersecurity, and risk management, and assist clients in evaluating and implementing risk management strategies for emerging AI technologies. For further discussions regarding AI implementation in the workplace, please contact Palmina M. Fava and Parker D. Hancock.
Key Contacts
Related Insights
- Insight
V&E Data Privacy Update
July 22, 2024
This information is provided by Vinson & Elkins LLP for educational and informational purposes only and is not intended, nor should it be construed, as legal advice.